IoT
hub and stream analytics are two very good feature provided by Azure cloud, and
here I am trying to showcase how we can use it for an IoT use case.
Steps
in high level
1. Create IoT Hub in Azure
2. Create devices
In
order to create the “IoT Hub”, first we need to login to the azure portal https://portal.azure.com with a valid id. (Free trials
will be expire usually within one month)
Once
you login you can see the below screen. You can ignore the blackened line as
they are created for different use.
Click on “New” and enter
“IoT Hub”
Use
“Create” button to move to the next blade.
Select
the “Pricing” as per your need but only one IoT hub can be created using “F1
Free”.
On
click on “Create” button the deployment of new IoT Hub will be starting and we
can see its status in the right top
1 Create device in IoT Hub
Click
on the newly created “IoT Hub” from “All resource” page and then navigate to Device
explorer
Once
succeeded, we can see the new created resource in “All Resource” page.
1. Create device in IoT Hub
Click
on the newly created “IoT Hub” from “All resource” page and then navigate to Device
explorer.
I
have created 5 devices for the trial, and it looks as below
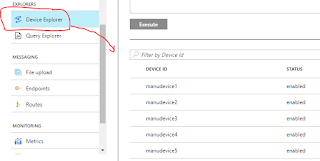
1 Create input in stream
analytics job
You
can see the “Input” link under Job topology of the Stream Analytics Job
Create output in stream
analytics job.
You
can see the “Output” link under Job topology of the Stream Analytics Job
In
order to filter and save two different type of critical errors, I have created
two output in this Stream analytic job and it looks are below.
In
order to filter and save two different type of critical errors, I have created
two output in this Stream analytic job and it looks are below.
1. Now we need to send simulated
data to the IoT hub devices and using a Stream Analytics query we will be able
to process it
To
send data I have used a sample Java code. In the Java application we need to
feed to the IoT hub full name and device’s primary key.
(Download
Sample java code)
Here
using the java application I am sending below values along with the device-ids.
At
the time of configuring the device/gateways we need to set the device-id based
on a plan and usually this will be unique to the system.
{"temperature":84,"heartbate":159,"bladeload":1267,"error_code":1,"deviceId":"manudevice4"}
After the data simulator, we can set the Query
for stream analytics and I have used the below simple query to filter the
records based on the error code to two different data set
-------------------
SELECT
deviceId as
deviceid,heartbate as heartbeat,temperature as temperature,bladeload as
bladeload,error_code as error_code,System.Timestamp as ts
INTO
manuoutputerror1devices
FROM
manuinput where error_code = 1
SELECT
deviceId as
deviceid,heartbate as heartbeat,temperature as temperature,bladeload as
bladeload,error_code as error_code,System.Timestamp as ts
INTO
manuoutputerror2devices
FROM
manuinput where error_code = 2
-------------------
Once
the query setup is over, we can start the Steam Analytics job and then we need
to execute the java program to send the data continuously, and then we will be
able to see two output files in two output location based on the query filter
we used in the Stream Analytics job.
Instead
of data as csv, we will be able to send the data to another layer for further
action without any delay.